AI as a Medical Device: Key Challenges and Future Directions
- Lloyd Price
- 10 minutes ago
- 10 min read
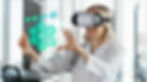
Exec Summary
Artificial Intelligence as a Medical Device (AIaMD) refers to AI-based software intended for medical purposes, such as diagnosis, treatment, monitoring, or prevention of disease, regulated as a medical device. Below is a concise overview of AIaMD, its regulatory landscape, challenges and considerations, based on current global frameworks.
Definition and Scope
AIaMD is a subset of Software as a Medical Device (SaMD), which includes software intended for medical purposes without being part of a hardware medical device. Examples include:
AI algorithms analysing MRI images to detect strokes.
Software predicting cardiac risks based on patient data.
Clinical decision support (CDS) tools aiding healthcare providers.
AIaMD is regulated when it meets the definition of a medical device, typically based on its intended use and risk level. Software for wellness (e.g., step counters) or administrative tasks is generally exempt.
Regulatory Frameworks
AIaMD regulation varies globally but focuses on safety, effectiveness, and risk management. Key regions include:
United States (FDA)
Regulation: The FDA regulates AIaMD as SaMD under pathways like 510(k) clearance, De Novo classification, or premarket approval, based on risk (Class I to III). AIaMD is often classified as Software as a Medical Device (SaMD).
Approach: The FDA uses a risk-based approach, focusing on intended use and patient risk if the software fails. Most AIaMD uses "locked algorithms" but adaptive AI requires a Predetermined Change Control Plan (PCCP) for updates.
Guidance: The FDA’s 2025 draft guidance addresses AIaMD lifecycle management, including bias, transparency, and post-market monitoring. Over 1,000 AI-enabled devices are FDA-authorised.
Challenges: Adaptive AI/ML systems challenge traditional regulatory paradigms, requiring premarket review for significant modifications.
European Union (EU)
Regulation: AIaMD is regulated under the Medical Devices Regulation (MDR) and In Vitro Diagnostic Medical Devices Regulation (IVDR). The EU AI Act (effective 2024) adds requirements for AI systems, particularly high-risk AIaMD.
Classification: AIaMD is classified by risk (Class I to III). High-risk AI systems (HRAIS) under the AI Act require notified body certification and GDPR compliance.
Compliance: Manufacturers must provide a single Declaration of Conformity for MDR/IVDR and AI Act, ensuring safety, performance, and data protection.
Challenges: The AI Act’s broad scope and overlap with MDR/IVDR create complexity. Adaptive AI and opacity raise concerns about trustworthiness and bias.
United Kingdom (MHRA)
Regulation: AIaMD is regulated under the UK Medical Devices Regulations 2002 (UK MDR 2002), with reforms via the Software and AI as a Medical Device Change Programme.
Approach: The MHRA emphasises patient safety, clear manufacturer requirements, and international harmonisation through the International Medical Device Regulators Forum (IMDRF). The AI Airlock sandbox pilots regulatory solutions.
Guidance: The MHRA provides guidance on SaMD and AIaMD, addressing transparency, adaptivity, and health inequalities.
Challenges: Balancing innovation with safety, especially for generative AI, requires updated regulations and public engagement.
Australia (TGA)
Regulation: AIaMD is regulated as SaMD under the Therapeutic Goods (Medical Devices) Regulations 2002, with a risk-based classification.
Approach: AIaMD for diagnosis, treatment, or monitoring is regulated, requiring clinical and technical evidence. Generative AI (e.g., LLMs) is regulated if used for medical purposes.
Challenges: Ensuring robust evidence for high-risk AIaMD and harmonising with global standards.
China (NMPA)
Regulation: The National Medical Products Administration (NMPA) regulates AIaMD with guidelines on deep learning, algorithm safety, and cybersecurity.
Approach: A rules-based system requires clinical evidence and lifecycle management. As of July 2023, 59 AI medical devices were approved.
Challenges: Language barriers limit global understanding, but China’s large data pools drive innovation.
Key Regulatory Considerations
Safety and Effectiveness:
Manufacturers must demonstrate diagnostic accuracy (e.g., sensitivity, specificity) and mitigate risks like bias or algorithm drift.
Post-market surveillance, including adverse event reporting (e.g., MHRA’s Yellow Card scheme), is mandatory.
Transparency and Bias:
AIaMD must be explainable to ensure trust. Bias from training data can lead to inaccurate outcomes, requiring robust validation.
The FDA and EU emphasise addressing bias throughout the device lifecycle.
Adaptivity:
Adaptive AI, which evolves with new data, challenges static regulatory models. The FDA’s PCCP and MHRA’s AI Airlock address this.
Data Protection:
GDPR (EU) and similar laws mandate secure handling of patient data. Non-compliance can invalidate AIaMD certifications.
Global Harmonisation:
The IMDRF and other forums aim to align standards on transparency, risk management, and clinical evaluation to reduce regulatory fragmentation.
Challenges
Complexity: Overlapping regulations (e.g., EU AI Act and MDR) create compliance burdens.
Bias and Fairness: AIaMD may underperform across diverse populations if training data is not representative.
Ethical Concerns: Autonomy, accountability, and patient trust require clear guidelines.
Innovation vs. Safety: Regulators must balance rapid AI advancements with patient protection.
Future Directions
Harmonised Standards: Global efforts (e.g., IMDRF) aim to unify AIaMD regulations, focusing on algorithm transparency and cybersecurity.
Generative AI: Emerging AI, like LLMs, requires new regulatory approaches to address unpredictability.
Patient-Centric Regulation: Public engagement and health equity are priorities, especially in the UK.
Real-World Evidence: Post-market data will refine AIaMD performance and safety assessments.
AIaMD holds transformative potential for healthcare but requires robust regulation to ensure safety and effectiveness. Global frameworks are evolving, with the FDA, EU, MHRA, TGA, and NMPA addressing unique AI challenges like adaptivity and bias.
Harmonisation, transparency, and patient-centric approaches will shape the future of AIaMD regulation. For developers, navigating these regulations is critical, and resources like the MHRA’s guidance or FDA’s draft documents provide essential support.
Nelson Advisors > HealthTech M&A
Nelson Advisors specialise in mergers, acquisitions and partnerships for Digital Health, HealthTech, Health IT, Healthcare Cybersecurity, Healthcare AI companies based in the UK, Europe and North America. www.nelsonadvisors.co.uk
We work with our clients to assess whether they should 'Build, Buy, Partner or Sell' in order to maximise shareholder value and investment returns. Email lloyd@nelsonadvisors.co.uk
Nelson Advisors regularly publish Healthcare Technology thought leadership articles covering market insights, trends, analysis & predictions @ https://www.healthcare.digital
We share our views on the latest Healthcare Technology mergers, acquisitions and partnerships with insights, analysis and predictions in our LinkedIn Newsletter every week, subscribe today! https://lnkd.in/e5hTp_xb
#HealthTech #DigitalHealth #HealthIT #NelsonAdvisors #Mergers #Acquisitions #Growth #Strategy #Cybersecurity #HealthcareAI #Partnerships #NHS #UK #Europe #USA #Canada
Nelson Advisors
Hale House, 76-78 Portland Place, Marylebone, London, W1B 1NT
Contact Us
Meet Us
Digital Health Rewired > 18-19th March 2025
NHS ConfedExpo > 11-12th June 2025
HLTH Europe > 16-19th June 2025
HIMSS AI in Healthcare > 10-11th July 2025
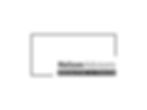
Key challenges of Artificial Intelligence as a Medical Device (AIaMD)
The key challenges of Artificial Intelligence as a Medical Device (AIaMD) revolve around ensuring safety, effectiveness, and ethical use while fostering innovation. Below is a concise list of the primary challenges, based on global regulatory frameworks and current insights:
Regulatory Complexity:
Overlapping regulations (e.g., EU AI Act and MDR/IVDR, FDA’s SaMD rules) create compliance burdens for manufacturers.
Adaptive AI, which evolves with data, challenges static regulatory models, requiring frameworks like the FDA’s Predetermined Change Control Plan (PCCP) or MHRA’s AI Airlock.
Bias and Fairness:
AIaMD trained on non-representative datasets can produce biased outcomes, leading to inaccurate diagnoses or treatments across diverse populations.
Mitigating bias requires robust validation and transparency throughout the device lifecycle.
Transparency and Explainability:
AI’s "black box" nature makes it hard to explain decision-making, undermining trust among healthcare providers and patients.
Regulators (e.g., FDA, MHRA) emphasise explainable AI to ensure accountability and clinical acceptance.
Safety and Effectiveness:
Ensuring diagnostic accuracy (e.g., sensitivity, specificity) and mitigating risks like algorithm drift or failure in real-world settings is critical.
Post-market surveillance, including adverse event reporting, is challenging for continuously learning systems.
Data Protection and Cybersecurity:
Compliance with data privacy laws (e.g., GDPR in the EU) is mandatory, as AIaMD often processes sensitive patient data.
Cybersecurity risks, such as hacking or data breaches, threaten patient safety and device integrity.
Ethical Concerns:
Issues like patient autonomy, accountability for AI errors, and equitable access to AIaMD raise ethical questions.
Public trust hinges on addressing these concerns through clear guidelines and engagement.
Global Harmonisation:
Divergent regulatory standards across regions (e.g., FDA, EU, NMPA, TGA) complicate global market access for AIaMD developers.
Efforts like the International Medical Device Regulators Forum (IMDRF) aim to align standards but are ongoing.
Balancing Innovation and Safety:
Rapid AI advancements, especially in generative AI, outpace regulatory frameworks, creating tension between innovation and patient protection.
Regulators must adapt to emerging technologies without stifling development.
Clinical Validation and Evidence:
Generating robust clinical evidence for AIaMD, especially for high-risk applications, is resource-intensive.
Real-world evidence collection is needed to monitor performance and safety post-market.
Adoption and Trust:
Healthcare providers may resist AIaMD due to concerns about reliability, liability, or job displacement.
Patient skepticism, fuelled by ethical and accuracy concerns, can hinder widespread adoption.
These challenges require collaboration among regulators, developers, and healthcare stakeholders to ensure AIaMD is safe, effective, and equitable.
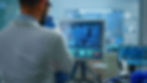
Future Directions of Artificial Intelligence as a Medical Device (AIaMD)
The future of Artificial Intelligence as a Medical Device (AIaMD) is poised for transformative growth, driven by technological advancements, regulatory evolution, and increasing integration into healthcare systems.
Below is a concise overview of key future directions, grounded in recent developments and trends:
1. Enhanced Diagnostic and Predictive Capabilities
Precision Diagnostics: AIaMD will continue to advance in analyzing multimodal data (e.g., imaging, genomics, electronic health records) to improve diagnostic accuracy for conditions like cancer, neurological disorders, and cardiovascular diseases. For instance, deep learning models are being refined to detect subtle patterns in medical imaging, such as early-stage diabetic retinopathy or ischemic stroke.
Predictive Analytics: AI will play a larger role in preventative medicine by predicting disease risks and complications. Examples include real-time monitoring of diabetic patients to suggest interventions or forecasting disease progression in chronic conditions like Parkinson’s.
Multimodal AI: Future AIaMD systems will integrate diverse data sources (e.g., radiology, lab values, and patient history) for holistic diagnostic reasoning, as seen in models like Google’s Med-PaLM M, which combines clinical language, imaging, and genomics.
2. Personalised Medicine and Treatment Optimisation
Tailored Therapies: AIaMD will enable hyper-personalised treatment plans by analysing individual patient data, such as genomic profiles, to recommend optimal drug therapies or interventions. This is already evident in oncology, where algorithms predict responses to chemotherapeutic drugs.
Drug Discovery: AI will accelerate drug development by identifying novel therapeutic targets and optimising clinical trial designs, particularly for rare diseases. Companies like Verge Genomics use machine learning to analyse genomic data for neurological disorders.
Real-Time Decision Support: AIaMD will provide clinicians with real-time recommendations, such as adjusting medication dosages or identifying surgical targets, enhancing precision in procedures like endoscopic surgeries.
3. Regulatory and Ethical Advancements
Adaptive Regulatory Frameworks: Regulatory bodies like the FDA and MHRA are developing frameworks to accommodate AIaMD’s dynamic nature. Predetermined Change Control Plans (PCCPs) allow pre-specified modifications to AI devices post-market, reducing the need for repeated approvals.
Global Harmonisation: Efforts are underway to standardise AIaMD regulations across jurisdictions. The MHRA, FDA, and Health Canada have established guiding principles for Good Machine Learning Practice (GMLP) and transparency to ensure safety and interoperability.
Ethical Considerations: Addressing algorithmic bias, ensuring transparency (e.g., explainable AI), and protecting patient privacy are critical. Federated learning, which trains models on decentralized data, is emerging to balance data access with privacy.
4. Integration into Clinical Workflows
Seamless Adoption: AIaMD will be embedded into routine clinical practice, enhancing workflows through tools like conversational AI, voice recognition, and visual overlays. This will reduce administrative burdens and allow clinicians to focus on patient care.
Software-Defined Devices: The shift toward software-defined medical devices, supported by platforms like NVIDIA’s Holoscan, will enable continuous updates and improvements, similar to smartphone apps.
Surgical and Procedural Support: AIaMD will assist in minimally invasive surgeries by providing real-time insights, as demonstrated by companies like Kaliber AI and Johnson & Johnson MedTech, which leverage AI for surgical analytics and visualisation.
5. Emerging Technologies and Trends
Generative AI: While not yet FDA-approved for clinical use, generative AI models (e.g., vision-language models) are being explored for drafting reports or simulating clinical scenarios, potentially streamlining documentation and training.
Explainable AI: To build trust, future AIaMD will prioritise interpretability, ensuring clinicians understand the rationale behind AI recommendations. This is critical for widespread adoption.
Continuous Monitoring: AIaMD will support ongoing patient monitoring, as seen in devices like Medtronic’s Guardian system for glucose monitoring, which pairs with smartphones for real-time data.
6. Challenges and Considerations
Data Privacy and Security: Patient data breaches and ownership disputes remain concerns. Solutions like blockchain-based Merkle trees for secure data exchange and anonymised NHS imaging databases (e.g., BRAIN) are being explored.
Algorithmic Bias: Ensuring AI models are trained on diverse datasets to avoid biased outcomes is essential, particularly for underrepresented populations.
Workforce Impact: AIaMD may automate routine tasks, raising concerns about job displacement. However, it is expected to augment rather than replace clinicians, requiring new skills like AI literacy.
Public Trust: Overcoming skepticism about AI’s reliability and maintaining the “human face of medicine” will be crucial for adoption.
7. Future Outlook (2025–2035)
Widespread Adoption: With over 1,000 FDA-authorised AI-enabled devices as of 2025, the number is expected to grow exponentially, driven by cloud computing and edge AI platforms.
AI-Augmented Healthcare: AIaMD will support the “quadruple aim” of healthcare—enhancing patient outcomes, reducing costs, improving clinician experience, and advancing health equity—through connected, precision-driven systems.
Educational Shifts: Medical curricula will evolve to include AI literacy, with programs like doctor-engineering degrees preparing clinicians to collaborate with AI systems.
AIaMD is set to revolutionise healthcare by enhancing diagnostics, personalising treatments, and streamlining clinical workflows. However, realizing its potential requires addressing regulatory, ethical, and technical challenges. Collaborative efforts between developers, regulators, clinicians, and patients will be key to ensuring AIaMD delivers safe, equitable, and effective solutions.
Nelson Advisors > HealthTech M&A
Nelson Advisors specialise in mergers, acquisitions and partnerships for Digital Health, HealthTech, Health IT, Healthcare Cybersecurity, Healthcare AI companies based in the UK, Europe and North America. www.nelsonadvisors.co.uk
We work with our clients to assess whether they should 'Build, Buy, Partner or Sell' in order to maximise shareholder value and investment returns. Email lloyd@nelsonadvisors.co.uk
Nelson Advisors regularly publish Healthcare Technology thought leadership articles covering market insights, trends, analysis & predictions @ https://www.healthcare.digital
We share our views on the latest Healthcare Technology mergers, acquisitions and partnerships with insights, analysis and predictions in our LinkedIn Newsletter every week, subscribe today! https://lnkd.in/e5hTp_xb
#HealthTech #DigitalHealth #HealthIT #NelsonAdvisors #Mergers #Acquisitions #Growth #Strategy #Cybersecurity #HealthcareAI #Partnerships #NHS #UK #Europe #USA #Canada
Nelson Advisors
Hale House, 76-78 Portland Place, Marylebone, London, W1B 1NT
Contact Us
Meet Us
Digital Health Rewired > 18-19th March 2025
NHS ConfedExpo > 11-12th June 2025
HLTH Europe > 16-19th June 2025
HIMSS AI in Healthcare > 10-11th July 2025
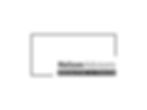