Are AI tools failing Women's Health?
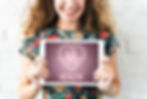
Exec Summary:
There are valid concerns about whether AI tools are adequately addressing women's health needs due to issues like bias, lack of research, and collaboration. Here's a breakdown:
Bias in Data:
Underrepresentation: Women have historically been underrepresented in medical research and clinical trials. This means that AI algorithms trained on these datasets may not accurately reflect the health needs and experiences of women.
Male-centric focus: Many medical studies and data collection efforts have focused primarily on male subjects. As a result, AI tools may be better at diagnosing and treating conditions that are more common in men, while overlooking or misinterpreting symptoms that are more prevalent in women.
Lack of diversity: Even when women are included in datasets, there may be a lack of diversity in terms of race, ethnicity, socioeconomic status, and other factors. This can lead to AI tools that are biased towards certain groups of women, while neglecting the needs of others.
Lack of Research:
Gender gap in research: There is a well-documented gender gap in medical research, with less funding and attention devoted to conditions that primarily affect women. This lack of research can hinder the development of AI tools that are specifically designed for women's health needs.
Limited understanding of female physiology: Women's bodies and hormonal systems are complex and dynamic. More research is needed to fully understand how these factors influence health and disease, and how AI can be used to personalise care for women at different stages of life.
'Without enough care, AI could potentially, in a not good way, incorporate all the same biases that have plagued our healthcare system for too long. There is already evidence of AI healthcare technologies working more effectively for men than for women.'
Source: Minister of State for Women's Health, Baroness Merron, spoke at the Responsible AI: Women and Healthcare Conference 2025, in London.
Lack of Collaboration:
Siloed approach: AI development in healthcare often occurs in silos, with limited collaboration between researchers, clinicians, and technology developers. This can lead to AI tools that are not well-integrated into clinical practice or that do not adequately address the needs of patients.
Need for interdisciplinary collaboration: Addressing the complex challenges in women's health requires collaboration across multiple disciplines, including medicine, engineering, computer science, and social sciences. This can help ensure that AI tools are developed and implemented in a way that is both technically sound and socially responsible.
Consequences and Solutions:
Misdiagnosis and delayed treatment: Biased AI tools can lead to misdiagnosis or delayed treatment for women, potentially resulting in worse health outcomes.
Exacerbating health disparities: If AI tools are not developed and implemented equitably, they can exacerbate existing health disparities between men and women, and between different groups of women.
Addressing the Challenges:
Improving data collection: Efforts are needed to collect more diverse and representative data on women's health, including data on specific conditions that disproportionately affect women.
Promoting research on women's health: Increased funding and attention are needed to support research on women's health, including studies that investigate the unique biological and social factors that influence women's health outcomes.
Fostering collaboration: Collaboration between researchers, clinicians, technology developers, and patients is essential to ensure that AI tools are developed and implemented in a way that is safe, effective, and equitable for all women.
By acknowledging and addressing these challenges, we can work towards a future where AI tools are used to improve women's health and reduce disparities in healthcare
Nelson Advisors
Healthcare Technology > Mergers, Acquisitions, Growth, Strategy, Investments http://www.nelsonadvisors.co.uk/
We work with Healthcare Technology founders, owners and investors to assess whether they should 'Build, Buy, Partner, Invest or Sell' in order to maximise shareholder value and investment returns. lloyd@nelsonadvisors.co.uk/
We regularly share our thoughts on Healthcare Technology mergers, acquisitions, growth, strategy, investments, market insights & predictions on our blog https://www.healthcare.digital
We publish a weekly LinkedIn Newsletter covering Healthcare Technology mergers, acquisitions, growth, strategy, investments, insights & predictions. Subscribe Today! https://lnkd.in/e5hTp_xb
#HealthTech #DigitalHealth #HealthIT #NelsonAdvisors #Mergers #Acquisitions #Growth #Strategy #Innovation #NHS #VentureCapital #PrivateEquity #UK #Europe
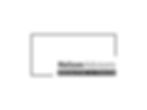
The role of AI in the future of women's health
Minister of State for Women's Health, Baroness Merron, spoke at the Responsible AI: Women and Healthcare Conference 2025, in London.
'Ss we look to AI, we need to ensure that 51% of our population must be worked with and for. This is not a minority group. We are a majority group and with particular healthcare needs. So, by taking steps to eliminate bias in healthcare AI, we will build trust, and I do think trust is so important, to build trust in this next wave of healthcare technologies and ensure that digital solutions can work for everyone.
We are, in government, committed to providing that support and enabling your efforts to come to fruition. We have supported the delivery of the Standing Together recommendations, which is a crucial piece of work developing standards for AI data sets, ensuring that they do reflect the diversity of the patient population and mean that we can see products that work for everybody.
With the National Institute for Health and Care Research, we are making sure that the UK research community incorporates sex and gender into its research, supporting the crucial work in the research inclusion strategy and finalising a sex and gender policy framework for funders through the Medical Science, Sex and Gender Equity project. But there is, of course, so much more to do and so much further that we can go to help you achieve the goal of making AI in healthcare work for everyone.
We will stand by your side in this crucial endeavour, and we are committed to enabling your efforts and finding ways to do that, because I believe it’s only with your expertise and your insight that the potential for digital transformation can be fully realised because what we want to see is faster diagnosis. We want to see better treatment. We want more efficient care to every person across the country.
Data biases are causing an issue for the use of AI in women's health
Data biases are a major stumbling block in the effective use of AI in women's health. Here's a breakdown of how this issue manifests and why it's so problematic:
Types of Data Bias Affecting Women's Health AI:
Underrepresentation: Women have historically been underrepresented in medical research and clinical trials. This means that AI algorithms trained on these datasets may not accurately reflect the health needs and experiences of women.
Male-centric focus: Many medical studies and data collection efforts have focused primarily on male subjects. As a result, AI tools may be better at diagnosing and treating conditions that are more common in men, while overlooking or misinterpreting symptoms that are more prevalent in women.
Lack of diversity: Even when women are included in datasets, there may be a lack of diversity in terms of race, ethnicity, socioeconomic status, and other factors. This can lead to AI tools that are biased towards certain groups of women, while neglecting the needs of others.
Consequences of Data Bias:
Misdiagnosis and delayed treatment: Biased AI tools can lead to misdiagnosis or delayed treatment for women, potentially resulting in worse health outcomes. For example, an AI algorithm trained primarily on male heart attack symptoms might miss or downplay atypical symptoms more common in women, leading to delayed diagnosis and treatment.
Exacerbating health disparities: If AI tools are not developed and implemented equitably, they can exacerbate existing health disparities between men and women, and between different groups of women. For instance, an AI tool for skin cancer detection trained primarily on lighter skin tones might be less accurate in diagnosing skin cancer in women with darker skin.
Reinforcing stereotypes: Biased AI tools can perpetuate harmful stereotypes and biases about women's health. For example, an AI chatbot designed to provide health information might inadvertently reinforce gender stereotypes about mental health or reproductive health.
Addressing Data Bias in Women's Health AI:
Improving data collection: Efforts are needed to collect more diverse and representative data on women's health, including data on specific conditions that disproportionately affect women. This includes ensuring representation across race, ethnicity, age, socioeconomic status, and other relevant factors.
Promoting research on women's health: Increased funding and attention are needed to support research on women's health, including studies that investigate the unique biological and social factors that influence women's health outcomes. This research can inform the development of more accurate and equitable AI tools.
Developing bias detection and mitigation techniques: Researchers need to develop methods for identifying and mitigating bias in datasets and AI algorithms. This includes techniques for data augmentation, re-sampling, and algorithm modification.
Fostering collaboration: Collaboration between researchers, clinicians, technology developers, and patients is essential to ensure that AI tools are developed and implemented in a way that is safe, effective, and equitable for all women. This includes involving women in the design and evaluation of AI tools to ensure that their needs and perspectives are taken into account.
The Bottom Line:
Data bias is a critical issue that must be addressed in order to ensure that AI tools are used effectively and equitably in women's health. By taking steps to improve data collection, promote research, develop bias mitigation techniques, and foster collaboration, we can work towards a future where AI helps to improve women's health outcomes and reduce disparities in healthcare.
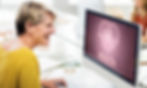
Lack of research and collaboration are causing an issue for the use of AI in women's health
The lack of robust research and meaningful collaboration are significant hurdles for the effective and equitable use of AI in women's health. Here's why:
Lack of Research:
Underfunding and Underrepresentation: Women's health research has historically been underfunded and underrepresented. This means that many conditions that primarily or disproportionately affect women are understudied, limiting our understanding of their causes, progression, and optimal treatments. This knowledge gap directly impacts the ability to develop effective AI tools.
Limited Understanding of Female Physiology: Women's bodies and hormonal systems are complex and dynamic, with fluctuations across different life stages (menstruation, pregnancy, menopause). More research is needed to fully understand how these factors influence health and disease, and how AI can be used to personalise care for women at different stages of life.
Data Gaps: There are significant gaps in data related to women's health, particularly for specific conditions that affect women disproportionately or differently than men. This lack of data makes it challenging to train AI algorithms that are accurate and reliable for women's health needs.
Lack of Collaboration:
Siloed Approach: AI development in healthcare often occurs in silos, with limited collaboration between researchers, clinicians, technology developers, and patients. This can lead to AI tools that are not well-integrated into clinical practice or that do not adequately address the needs of patients.
Need for Interdisciplinary Collaboration: Addressing the complex challenges in women's health requires collaboration across multiple disciplines, including medicine, engineering, computer science, and social sciences. This can help ensure that AI tools are developed and implemented in a way that is both technically sound and socially responsible.
Patient Involvement: Women need to be actively involved in the design, development, and evaluation of AI tools for their health. This can help ensure that the tools are relevant to their needs, address their concerns, and are user-friendly.
Consequences:
Ineffective AI Tools: Without adequate research and collaboration, AI tools may not be effective in addressing the specific health needs of women, leading to inaccurate diagnoses, inappropriate treatments, and potentially harmful outcomes.
Exacerbating Health Disparities: If AI tools are not developed and implemented equitably, they can exacerbate existing health disparities between men and women, and between different groups of women.
Erosion of Trust: If women do not trust that AI tools are being developed and used in a way that is in their best interests, they may be less likely to adopt and benefit from these technologies.
Addressing the Challenges:
Prioritising Women's Health Research: Increased funding and attention are needed to support research on women's health, including studies that investigate the unique biological and social factors that influence women's health outcomes.
Fostering Collaboration: Collaboration between researchers, clinicians, technology developers, and patients is essential to ensure that AI tools are developed and implemented in a way that is safe, effective, and equitable for all women.
Promoting Diversity and Inclusion: Efforts are needed to ensure that the teams developing AI tools for women's health are diverse and inclusive, reflecting the diversity of the women they are intended to serve.
Establishing Ethical Guidelines: Clear ethical guidelines are needed to ensure that AI tools for women's health are developed and used in a responsible and ethical manner, protecting patient privacy and ensuring fairness and transparency.
By addressing these challenges, we can work towards a future where AI tools are used to improve women's health and reduce disparities in healthcare.
Positive future for use of AI in women's health
Despite the challenges, the future of AI in women's health and FemTech is bright. Here's why:
1. Growing Recognition and Investment:
Increased Awareness: There's a growing awareness of the need to address the historical biases and gaps in women's health research and care. This is leading to increased attention and investment in AI and FemTech solutions specifically designed for women's health needs.
Funding and Support: Venture capital and other funding sources are increasingly recognising the potential of FemTech and AI in women's health, leading to more resources for research, development, and implementation.
2. Technological Advancements:
Improved AI Algorithms: Advances in AI and machine learning are leading to more sophisticated algorithms that can analyse complex data and provide personalised insights for women's health.
Wearable Technology: The rise of wearable devices and sensors provides a wealth of data that can be used to track women's health metrics and personalise interventions.
Telehealth and Remote Monitoring: AI-powered telehealth platforms can improve access to care for women, especially those in remote areas or with limited mobility.
3. Expanding Applications:
Menstrual Health: AI-powered apps and devices can help women track their menstrual cycles, predict ovulation, and manage menstrual-related symptoms.
Fertility and Family Planning: AI can assist with fertility tracking, personalised family planning, and even support in-vitro fertilisation (IVF) processes.
Pregnancy and Maternal Health: AI can be used to monitor pregnancy, detect potential complications, and provide personalised support to expectant mothers.
Menopause Management: AI-powered tools can help women navigate the menopausal transition, manage symptoms, and access personalised information and support.
Mental Health: AI chatbots and virtual assistants can provide personalised mental health support and therapy for women, addressing issues like postpartum depression and anxiety.
Chronic Conditions: AI can assist in the management of chronic conditions that disproportionately affect women, such as endometriosis, polycystic ovary syndrome (PCOS), and autoimmune diseases.
4. Collaboration and Advocacy:
Interdisciplinary Collaboration: There's a growing emphasis on interdisciplinary collaboration between researchers, clinicians, technology developers, and patients to ensure that AI tools are developed and implemented in a way that is safe, effective, and equitable for women.
Patient Advocacy: Women's health advocates and organisations are playing a crucial role in raising awareness of the need for AI solutions that address the specific needs of women and in ensuring that these technologies are developed and used responsibly.
5. Potential Benefits:
Improved Diagnostics: AI can assist in the early detection and diagnosis of conditions that affect women, leading to better outcomes.
Personalised Treatment: AI can help tailor treatments to individual women based on their unique characteristics, preferences, and needs.
Enhanced Access: AI-powered telehealth and remote monitoring can improve access to care for women, especially those in underserved areas.
Empowered Women: AI tools can empower women to take control of their health by providing them with personalised information, insights, and support.
Looking Ahead:
The future of AI in women's health and FemTech is full of promise. By continuing to address the challenges related to data bias, research gaps, and collaboration, we can unlock the full potential of AI to improve women's health outcomes and reduce disparities in healthcare.
Nelson Advisors
Healthcare Technology > Mergers, Acquisitions, Growth, Strategy, Investments http://www.nelsonadvisors.co.uk/
We work with Healthcare Technology founders, owners and investors to assess whether they should 'Build, Buy, Partner, Invest or Sell' in order to maximise shareholder value and investment returns. lloyd@nelsonadvisors.co.uk/
We regularly share our thoughts on Healthcare Technology mergers, acquisitions, growth, strategy, investments, market insights & predictions on our blog https://www.healthcare.digital
We publish a weekly LinkedIn Newsletter covering Healthcare Technology mergers, acquisitions, growth, strategy, investments, insights & predictions. Subscribe Today! https://lnkd.in/e5hTp_xb
#HealthTech #DigitalHealth #HealthIT #NelsonAdvisors #Mergers #Acquisitions #Growth #Strategy #Innovation #NHS #VentureCapital #PrivateEquity #UK #Europe
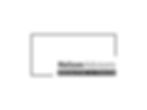