ROI on Healthcare AI: Return on Influence
- Lloyd Price
- 10 hours ago
- 11 min read
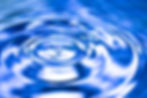
Exec Summary
The concept of "return on influence" (ROI) in healthcare AI shifts the focus from purely financial metrics (return on investment) to broader, non-monetary impacts that shape stakeholder behavior and system outcomes. Below, we explore how AI in healthcare influences retention, satisfaction, access, and trust, drawing on available insights and critical analysis.
Retention
AI influences patient and clinician retention by enhancing care delivery and operational efficiency, fostering loyalty to healthcare providers or systems.
Patients: AI-driven tools, like automated appointment reminders, follow-up scheduling, and personalised care plans, reduce patient churn. For instance, predictive analytics can identify patients at risk of disengaging (e.g., missing appointments) and trigger interventions like tailored outreach. A 2018 article noted that simple strategies, such as automated reactivation campaigns, improve patient retention by ensuring follow-ups, particularly for chronic conditions.
Influence: By reducing barriers to care continuity, AI builds stronger patient-provider relationships, encouraging patients to stay within a practice. Over 60% of patients prefer digital booking options, and enabling these can prevent loss to competitors.
Clinicians: AI reduces burnout by streamlining workflows (e.g., automating documentation or triage), which can improve job satisfaction and retention. However, over-reliance on AI or perceived threats to professional autonomy may alienate clinicians, potentially reducing retention if not addressed.
Critical Perspective: While AI can improve retention, its effectiveness depends on seamless integration into workflows. Poorly designed systems or lack of clinician buy-in can backfire, leading to frustration and turnover. Retention gains are also uneven, as marginalized communities may not benefit equally if AI systems perpetuate biases.
Satisfaction
AI enhances satisfaction by personalising experiences and improving efficiency, but its impact hinges on transparency and usability.
Patients: AI-powered chatbots and virtual assistants provide 24/7 support, answer queries, and guide patients through care processes, boosting satisfaction. For example, AI self-diagnosis chatbots improve perceived service quality when they offer explainable, high-quality information. Over 60% of healthcare CFOs report using generative AI for real-time customer service, which correlates with positive patient feedback.
Influence: Patients feel valued when AI delivers timely, relevant interactions, such as personalised health tips or easy appointment access. However, satisfaction drops if AI feels impersonal or fails to address complex needs (e.g., lack of human empathy in robotic carers).
Clinicians: AI decision support tools, like diagnostic aids in radiology, enhance confidence in clinical decisions when reliable and transparent. However, black-box models or inconsistent performance can frustrate clinicians, lowering satisfaction.
Critical Perspective: Satisfaction is not universal. Patients valuing personal interaction may distrust AI-driven care, particularly in sensitive areas like surgery or mental health. Clinicians may resent AI if it disrupts established roles or increases liability fears (e.g., responsibility for AI errors). Satisfaction also varies by demographic, with older or less tech-savvy patients reporting lower comfort with AI.
Access
AI expands access to healthcare by overcoming logistical, geographic, and resource barriers, but disparities persist.
Patients: AI enables telemedicine, remote monitoring, and self-diagnosis tools, making care accessible to underserved or rural populations. FinTech platforms powered by AI improve financial access by optimising payment plans and broadening service reach. AI chatbots act as ubiquitous points of contact, empowering users to make health decisions without immediate clinician involvement.
Influence: By reducing wait times and enabling digital care delivery, AI makes healthcare more inclusive. For example, AI-driven triage systems prioritise urgent cases, improving access to timely interventions.
Systemic Impact: AI optimises resource allocation (e.g., predicting staffing needs or equipment shortages), indirectly improving access to care. Big data analytics enhance healthcare financing, ensuring sustainable service provision.
Critical Perspective: Access gains are uneven. AI models trained on biased or homogenous datasets may misallocate resources, favoring privileged groups (e.g., white patients receiving more referrals despite similar needs). Limited digital infrastructure in low-income areas or among marginalized communities restricts AI’s reach. Privacy concerns also deter some patients from using AI tools, reducing effective access.
Trust
Trust is the cornerstone of AI’s influence in healthcare, shaping adoption and effectiveness. It’s dynamic, context-dependent, and fragile.
Patients: Trust in AI depends on perceived reliability, transparency, and anthropomorphism. Studies show patients trust AI more when it mimics human-like interactions or provides clear explanations (e.g., in chatbots for self-diagnosis). However, distrust arises from opaque “black-box” systems, past AI failures, or cultural skepticism, particularly in marginalized communities where biased AI outputs have eroded confidence. Reduced communication during AI implementation also lowers patient trust.
Influence: Trust drives willingness to use AI tools, influencing adherence to AI-guided recommendations (e.g., medication reminders). Strong trust can amplify the placebo effect, enhancing perceived outcomes.
Clinicians: Clinicians trust AI when it’s reliable, controllable, and aligns with their expertise. Factors like transparency, past performance, and training quality shape trust. However, liability concerns (e.g., being held accountable for AI errors) and automation bias (over-reliance on AI) undermine trust. Clinicians in high-risk fields like surgery trust AI less than in radiology or dermatology due to perceived risks.
Influence: Trust determines whether clinicians adopt AI or revert to traditional methods. Appropriate trust, calibrated to AI’s purpose and performance, improves decision-making without compromising autonomy.
Critical Perspective: Trust is not a given. The absence of clear governance, ethical standards, or legal frameworks for AI fuels skepticism. Clinicians and patients alike fear bias, privacy breaches, and unaccountable failures. For example, AI trained on skewed data may produce biased outcomes, eroding trust among underrepresented groups. Cultural factors, like reliance on interpersonal relationships, further complicate trust in AI, especially in healthcare settings valuing human connection.
Return on Influence: A Holistic View
Unlike traditional ROI, which focuses on financial gains (e.g., cost savings from AI radiology platforms), return on influence measures AI’s ability to shape behaviors, perceptions, and system dynamics. AI’s influence manifests in:
Behavioural Shifts: Patients engage more with care (retention, adherence) when AI feels trustworthy and accessible. Clinicians adopt AI when it enhances, rather than threatens, their roles.
Systemic Change: AI fosters equitable access and resource allocation when designed responsibly, but biases or poor implementation can exacerbate inequities.
Cultural Impact: Trust and satisfaction build a culture of AI acceptance, but failures or opacity can entrench resistance, slowing adoption.
However, influence is harder to quantify than investment returns. Metrics like patient retention rates, satisfaction scores, access disparities, and trust surveys are needed but often lack standardization. The 90% of healthcare executives reporting positive ROI from generative AI suggest influence translates to tangible outcomes, but only when supported by robust infrastructure and governance.
Challenges and Recommendations
Challenges:
Bias and Equity: AI can perpetuate disparities if trained on biased data, undermining trust and access for marginalized groups.
Transparency: Opaque AI systems reduce trust and satisfaction, particularly among clinicians and patients valuing human interaction.
Liability and Governance: Unclear accountability for AI errors hinders clinician trust and adoption.
Digital Divide: Limited technological infrastructure in underserved areas restricts AI’s influence on access.
Recommendations:
Foster Appropriate Trust: Design AI with transparency (e.g., explainable outputs) and monitor performance metrics like calibration to ensure reliability.
Prioritize Equity: Use diverse datasets and regular audits to mitigate bias, ensuring equitable access and trust.
Engage Stakeholders: Involve clinicians, patients, and ethicists in AI design to align with cultural and professional values, enhancing satisfaction and retention.
Strengthen Governance: Establish clear legal and ethical frameworks to address liability and privacy, boosting trust.
Invest in Infrastructure: Scale AI beyond pilots with robust digital systems to maximize access and influence.
AI’s return on influence in healthcare lies in its ability to enhance retention, satisfaction, access, and trust, driving behavioral and systemic change. While financial ROI is critical (e.g., 90% of executives see positive returns), influence shapes long-term adoption and impact. By addressing challenges like bias, opacity, and governance, healthcare AI can maximize its influence, ensuring equitable, trusted, and accessible care. Critical scrutiny of implementation and stakeholder engagement will be key to realising this potential.
Nelson Advisors > HealthTech M&A
Nelson Advisors specialise in mergers, acquisitions and partnerships for Digital Health, HealthTech, Health IT, Healthcare Cybersecurity, Healthcare AI companies based in the UK, Europe and North America. www.nelsonadvisors.co.uk
We work with our clients to assess whether they should 'Build, Buy, Partner or Sell' in order to maximise shareholder value and investment returns. Email lloyd@nelsonadvisors.co.uk
Nelson Advisors regularly publish Healthcare Technology thought leadership articles covering market insights, trends, analysis & predictions @ https://www.healthcare.digital
We share our views on the latest Healthcare Technology mergers, acquisitions and partnerships with insights, analysis and predictions in our LinkedIn Newsletter every week, subscribe today! https://lnkd.in/e5hTp_xb
#HealthTech #DigitalHealth #HealthIT #NelsonAdvisors #Mergers #Acquisitions #Growth #Strategy #Cybersecurity #HealthcareAI #Partnerships #NHS #UK #Europe #USA #Canada
Nelson Advisors
Hale House, 76-78 Portland Place, Marylebone, London, W1B 1NT
Contact Us
Meet Us
Digital Health Rewired > 18-19th March 2025
NHS ConfedExpo > 11-12th June 2025
HLTH Europe > 16-19th June 2025
HIMSS AI in Healthcare > 10-11th July 2025
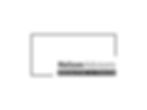
The ripple effect and return on influence in healthcare AI
The return on influence (ROI) in healthcare AI refers to the cascading, non-financial impacts of AI on stakeholders, systems, and society, as opposed to traditional return on investment focused on monetary gains. The ripple effect describes how AI’s influence spreads across interconnected domains, starting with direct users (patients, clinicians) and radiating to organisations, communities, and broader healthcare ecosystems.
Below, we explore how AI’s ripple effect shapes retention, satisfaction, access, and trust, emphasising the amplifying and interconnected nature of its influence.
The Ripple Effect of Healthcare AI
AI’s influence creates a chain reaction, where initial impacts (e.g., improved patient access) trigger secondary and tertiary effects (e.g., better health outcomes, reduced system costs). This ripple effect operates at multiple levels:
Individual Level: AI directly affects patients and clinicians through personalised care, streamlined workflows, or enhanced decision-making.
Organisational Level: Improved individual outcomes (e.g., higher satisfaction) boost operational efficiency, staff retention, and patient loyalty.
Community Level: Enhanced access and trust in AI-driven care reduce disparities and improve public health.
Systemic Level: Widespread adoption of AI reshapes healthcare financing, policy, and infrastructure, influencing entire ecosystems.
Each ripple amplifies AI’s return on influence, creating feedback loops that either reinforce positive outcomes or exacerbate challenges if mismanaged.
Return on Influence Across Key Domains
Retention: AI tools like predictive analytics identify patients at risk of disengaging and trigger personalised interventions (e.g., automated reminders, tailored care plans). For clinicians, AI reduces burnout by automating administrative tasks (e.g., EHR documentation), improving job satisfaction.
Example: AI-driven scheduling systems reduce no-shows by 20-30%, retaining patients within a practice.
Ripple Effect:
Patients: Retained patients build long-term relationships, increasing adherence to treatment and reducing emergency visits, which lowers costs for providers.
Clinicians: Lower turnover reduces recruitment costs and preserves institutional knowledge, enhancing care quality.
Systemic: Higher retention stabilises healthcare organisations, enabling reinvestment in AI and infrastructure, which further improves retention.
Influence Amplified: Loyal patients and clinicians advocate for AI-driven systems, spreading adoption and reinforcing organisational trust in AI investments.
Challenges: Poorly designed AI (e.g., overly complex interfaces) can frustrate users, reducing retention. Biased algorithms may neglect marginalized patients, weakening retention in underserved communities.
Satisfaction
Direct Impact: AI enhances patient satisfaction through 24/7 chatbots, personalized health recommendations, and reduced wait times. Clinicians benefit from decision support tools (e.g., AI diagnostics in radiology) that boost confidence when transparent and reliable.
Example: Over 60% of patients report higher satisfaction with AI-enabled digital booking or virtual consultations.
Ripple Effect:
Patients: Satisfied patients are more likely to adhere to AI-guided recommendations, improving health outcomes and reducing readmissions.
Clinicians: Higher satisfaction reduces burnout, fostering a positive workplace culture that attracts talent.
Community: Positive patient experiences shared via word-of-mouth or social platforms (e.g., X posts) enhance provider reputation, drawing more patients.
Systemic: High satisfaction drives demand for AI solutions, encouraging innovation and competition among vendors, which improves technology quality.
Influence Amplified: Satisfied stakeholders become AI advocates, accelerating adoption and shaping positive narratives around AI’s role in care.
Challenges: Opaque AI systems or lack of human-like interaction (e.g., robotic carers) can lower satisfaction, particularly for older patients or those valuing personal touch. Clinician frustration with unreliable AI can ripple into distrust across teams.
Access: AI expands access through telemedicine, remote monitoring, and AI-driven triage, reaching underserved or rural populations. AI-powered FinTech platforms optimize payment plans, improving financial access.
Example: AI chatbots provide instant health guidance, reducing barriers for those unable to visit clinics.
Ripple Effect:
Patients: Increased access leads to earlier interventions, reducing chronic disease burdens and healthcare costs.
Community: Equitable access narrows health disparities, improving public health metrics like life expectancy or infant mortality.
Organisational: Efficient resource allocation (e.g., AI predicting staffing needs) ensures more patients are served, enhancing provider capacity.
Systemic: Broadened access informs policy, driving investments in digital infrastructure and AI scalability.
Influence Amplified: As access improves, communities trust healthcare systems more, increasing
engagement with AI tools and creating a virtuous cycle of adoption and innovation.
Challenges: The digital divide (e.g., lack of internet in rural areas) limits AI’s reach. Biased AI models may prioritise privileged groups, exacerbating inequities and reducing effective access for marginalized populations.
Trust: Trust in AI hinges on reliability, transparency, and cultural alignment. Patients trust explainable AI (e.g., chatbots with clear reasoning), while clinicians trust tools that complement their expertise without threatening autonomy.
Example: Transparent AI diagnostics in dermatology build clinician trust, increasing adoption rates.
Ripple Effect:
Patients: Trust encourages adherence to AI recommendations, improving outcomes and reinforcing confidence in providers.
Clinicians: Trusted AI integration enhances collaboration, reducing errors and improving care quality.
Community: High trust in AI-driven care fosters public acceptance, reducing skepticism and encouraging participation in digital health initiatives.
Systemic: Widespread trust attracts investment in AI governance and ethical standards, ensuring long-term sustainability.
Influence Amplified: Trusted AI creates a cultural shift toward technology acceptance, influencing policy, education, and workforce training to prioritise AI literacy.
Challenges: Black-box AI, biased outcomes, or privacy breaches erode trust, with ripple effects like reduced adoption, legal challenges, and public backlash. Cultural skepticism, especially in communities with histories of medical mistrust, amplifies these risks.
Quantifying Return on Influence
Unlike financial ROI, return on influence is measured through qualitative and indirect metrics:
Retention: Patient return rates, clinician turnover rates, no-show reductions.
Satisfaction: Net Promoter Scores, patient feedback surveys, clinician morale indices.
Access: Telehealth adoption rates, reduction in care disparities, patient volume in underserved areas.
Trust: Trust indices (e.g., surveys on AI reliability), adoption rates of AI tools, social sentiment
These metrics are interconnected: high trust boosts satisfaction, which improves retention, which enhances access. The ripple effect ensures that influence in one domain amplifies others, creating exponential impact when managed well.
Critical Considerations
Positive Ripples:
Network Effects: As more stakeholders adopt AI, its value grows (e.g., larger datasets improve AI accuracy, benefiting all users).
Cultural Shifts: Successful AI implementations normalise technology in healthcare, influencing younger generations to expect digital-first care.
Economic Spillovers: Improved health outcomes reduce societal costs (e.g., fewer sick days), indirectly boosting economies.
Negative Ripples:
Bias Amplification: Biased AI can worsen inequities, eroding trust and access in vulnerable communities, with long-term societal costs.
Resistance Loops: Clinician or patient distrust can spread, slowing AI adoption and innovation.
Over-Reliance Risks: Automation bias or over-dependence on AI may degrade human skills, creating systemic vulnerabilities.
Mitigating Risks:
Ethical Design: Use diverse datasets and transparent algorithms to ensure equity and trust.
Stakeholder Engagement: Co-design AI with patients and clinicians to align with cultural and professional needs.
Governance: Establish clear accountability for AI errors to maintain trust and mitigate liability concerns.
Infrastructure Investment: Bridge the digital divide to ensure equitable access and maximize positive ripples.
Real-World Context
Industry Insights: Over 90% of healthcare executives report positive ROI from generative AI, suggesting that influence (e.g., satisfaction, access) translates to tangible outcomes. For example, AI radiology platforms improve diagnostic speed, influencing clinician satisfaction and patient trust.
Public Sentiment: social media posts reveal mixed views—some praise AI for accessibility (e.g., telehealth during pandemics), while others criticise biases or privacy risks, highlighting trust’s role in adoption.
Policy Trends: Governments are investing in AI governance (e.g., EU’s AI Act), recognizing its systemic ripple effects on trust and equity.
The return on influence in healthcare AI, amplified by its ripple effect, transforms retention, satisfaction, access, and trust into interconnected drivers of change. Starting with individual experiences, AI’s influence cascades to organisations, communities, and systems, creating feedback loops that either scale benefits or amplify risks. By prioritising transparency, equity, and stakeholder engagement, healthcare AI can maximise positive ripples, improving outcomes, reducing disparities, and fostering a culture of trust. However, biases, mistrust, or uneven access can trigger negative ripples, underscoring the need for responsible design and governance.
Nelson Advisors > HealthTech M&A
Nelson Advisors specialise in mergers, acquisitions and partnerships for Digital Health, HealthTech, Health IT, Healthcare Cybersecurity, Healthcare AI companies based in the UK, Europe and North America. www.nelsonadvisors.co.uk
We work with our clients to assess whether they should 'Build, Buy, Partner or Sell' in order to maximise shareholder value and investment returns. Email lloyd@nelsonadvisors.co.uk
Nelson Advisors regularly publish Healthcare Technology thought leadership articles covering market insights, trends, analysis & predictions @ https://www.healthcare.digital
We share our views on the latest Healthcare Technology mergers, acquisitions and partnerships with insights, analysis and predictions in our LinkedIn Newsletter every week, subscribe today! https://lnkd.in/e5hTp_xb
#HealthTech #DigitalHealth #HealthIT #NelsonAdvisors #Mergers #Acquisitions #Growth #Strategy #Cybersecurity #HealthcareAI #Partnerships #NHS #UK #Europe #USA #Canada
Nelson Advisors
Hale House, 76-78 Portland Place, Marylebone, London, W1B 1NT
Contact Us
Meet Us
Digital Health Rewired > 18-19th March 2025
NHS ConfedExpo > 11-12th June 2025
HLTH Europe > 16-19th June 2025
HIMSS AI in Healthcare > 10-11th July 2025
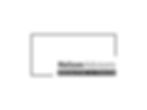