Small Language Models (SLMs) have the potential to revolutionise Healthcare AI in addition to LLMs

Exec Summary:
Small Language Models (SLMs) are a type of artificial intelligence (AI) that can process and generate human language. They are called "small" because they have fewer parameters (internal variables) than Large Language Models (LLMs). This makes them more efficient and easier to train, but also limits their capabilities.
SLMs use a similar approach to LLMs, but with a smaller scale. They are trained on massive amounts of text data, learning to predict the next word in a sequence. This allows them to generate human-like text, translate languages, write different kinds of creative content, and answer your questions in an informative way.
Small Language Models (SLMs) are a type of artificial intelligence that can process, understand, and generate human language. Here's a breakdown:
Size Matters: SLMs are significantly smaller than their larger counterparts, known as Large Language Models (LLMs). This size difference translates to:
Fewer Parameters: SLMs have a much smaller number of parameters, which are the internal variables that the model learns during training.
Reduced Computational Needs: This smaller size makes SLMs more efficient, requiring less computing power and memory to operate.
Key Advantages:
Efficiency: SLMs can run on devices with limited resources, such as smartphones and wearables.
Accessibility: This makes them more accessible to a wider range of developers and organisations.
Customisation: SLMs can be more easily fine-tuned for specific tasks and domains.
Applications:
On-device AI: SLMs can power AI features directly on your devices, enabling faster responses and offline capabilities.
Resource-constrained environments: They are suitable for applications where computational power is limited.
Specific tasks: SLMs can be tailored for niche applications like customer service chatbots or personalised language assistants.
In essence, SLMs offer a balance of power and efficiency, making them a valuable tool for a wide range of language-based applications.
Small Language Models (SLMs) have the potential to revolutionise healthcare AI in several ways:
Improved Accessibility and Efficiency: SLMs are smaller and less computationally demanding than larger models. This makes them easier to deploy on devices with limited resources, such as smartphones and wearables. This accessibility can lead to more widespread adoption of AI-powered healthcare solutions.
Enhanced Personalisation: SLMs can be trained on specific datasets, allowing them to provide personalised insights and recommendations based on individual patient data. This can lead to more tailored treatment plans and improved patient outcomes.
Streamlined Clinical Workflows: SLMs can automate tasks such as data entry, appointment scheduling, and medical coding, freeing up healthcare professionals to focus on patient care.
Improved Patient Engagement: SLMs can be used to create interactive chatbots and virtual assistants that can provide patients with information, answer their questions, and even monitor their health remotely. This can improve patient engagement and adherence to treatment plans.
Drug Discovery and Development: SLMs can accelerate drug discovery and development by analyzing vast amounts of data to identify potential drug candidates and predict their 1 efficacy and safety.
Precision Medicine: SLMs can help identify genetic and environmental factors that contribute to individual health risks, enabling the development of personalised prevention and treatment strategies.
However, it's important to note that SLMs also have limitations. They may not be as accurate or comprehensive as larger models, and they may require careful validation and testing to ensure their reliability and safety in healthcare settings.
Overall, SLMs have the potential to transform healthcare AI by making it more accessible, efficient, and personalised. As the technology continues to evolve, we can expect to see even more innovative applications of SLMs in the healthcare industry.
Nelson Advisors work with Healthcare Technology Founders, Owners and Investors to assess whether they should 'Build, Buy, Partner or Sell' in order to maximise shareholder value > https://nelsonadvisors.co.uk/
Healthcare Technology Thought Leadership from Nelson Advisors – Market Insights, Analysis & Predictions. Visit https://www.healthcare.digital
Buy Side, Sell Side, Growth & Strategy services for HealthTech Founders, Owners and Investors. Email lloyd@nelsonadvisors.co.uk
Nelson Advisors Healthcare Technology Newsletter > Mergers, Acquisitions, Growth, Strategy, Insights & Predictions. Subscribe Today! https://lnkd.in/e5hTp_xb
#HealthTech #DigitalHealth #HealthIT #NelsonAdvisors #Mergers #Acquisitions #Growth #Strategy #Innovation #NHS #VentureCapital #PrivateEquity #UK #Europe
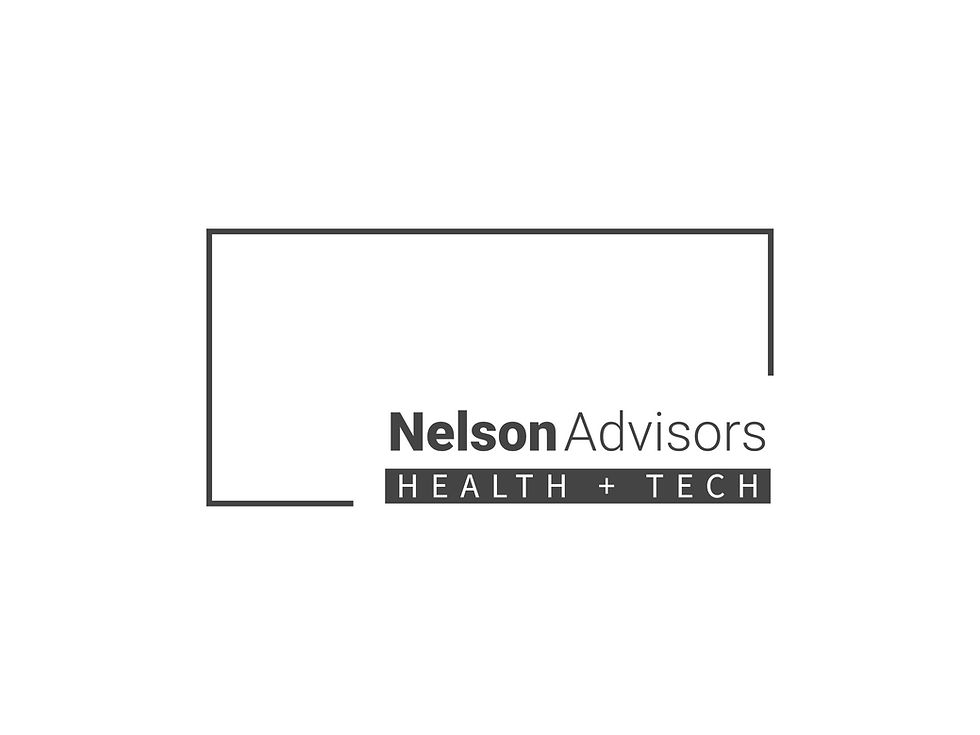
Small Language Models (SLMs) potential to revolutionise healthcare AI
Small Language Models (SLMs) have the potential to revolutionise healthcare AI in several ways:
Improved Clinical Decision Support: SLMs can analyze vast amounts of medical literature and patient data to provide doctors with real-time, evidence-based insights during consultations. This can help reduce diagnostic errors, improve treatment plans, and enhance overall patient care.
Personalised Medicine: SLMs can analyse individual patient data, including genetics, lifestyle, and medical history, to predict disease risk, tailor treatment plans, and recommend personalised interventions.
Drug Discovery and Development: SLMs can accelerate drug discovery by analyzing vast amounts of research data to identify potential drug targets, predict drug interactions, and optimize clinical trial design.
Mental Health Support: SLMs can be used to develop chatbots and virtual assistants that provide mental health support, such as cognitive behavioural therapy (CBT) techniques and emotional support.
Remote Patient Monitoring: SLMs can analyse data from wearable devices and other remote monitoring systems to identify potential health risks and alert healthcare providers to any concerning changes in a patient's condition.
Administrative Efficiency: SLMs can automate administrative tasks, such as scheduling appointments, managing medical records, and processing insurance claims, 1 freeing up healthcare professionals to focus on patient care.
Key Advantages of SLMs in Healthcare:
Efficiency: SLMs are smaller and faster to train than larger models, making them more suitable for real-time applications in healthcare settings.
Resource Efficiency: SLMs require less computational power and memory, making them more accessible for deployment in resource-constrained environments.
Privacy and Security: SLMs can be trained and deployed on-device or locally, reducing concerns about data privacy and security.
Explainability: SLMs can be more easily interpreted and understood than larger models, which is crucial for building trust and ensuring ethical use in healthcare.
Challenges and Considerations:
Data Quality and Bias: The accuracy and fairness of SLMs depend heavily on the quality and representativeness of the data they are trained on.
Ethical Considerations: It is crucial to address ethical concerns related to data privacy, algorithmic bias, and the potential for job displacement in the healthcare workforce.
Regulatory Compliance: The use of AI in healthcare must comply with relevant regulations and standards to ensure patient safety and data security.
Despite these challenges, SLMs have the potential to transform healthcare by improving patient outcomes, increasing efficiency, and reducing costs. Continued research and development in this area will be crucial to unlocking the full potential of SLMs in revolutionising healthcare AI.
Five examples of small language models in healthcare AI
Clinical Decision Support:
Example: An SLM trained on a specific disease (e.g., diabetes) can analyze patient data (medical history, lab results, lifestyle) and provide real-time recommendations to doctors on treatment options, medication adjustments, and potential complications.
Benefit: Improves diagnostic accuracy, personalises treatment plans, and enhances patient outcomes.
Medical Text Summarisation:
Example: An SLM can quickly summarize lengthy medical articles, research papers, and patient records, allowing doctors to efficiently grasp key information and make informed decisions.
Benefit: Saves time for healthcare providers, improves knowledge access, and facilitates better communication among healthcare professionals.
Mental Health Support Chatbots:
Example: An SLM-powered chatbot can engage in basic conversations with patients, provide emotional support, and offer simple cognitive behavioral therapy techniques for conditions like anxiety and depression.
Benefit: Increases accessibility to mental healthcare, especially in underserved areas, and provides initial support before professional intervention.
Drug Discovery:
Example: An SLM can analyze vast amounts of scientific literature to identify potential drug targets, predict drug interactions, and accelerate the drug discovery process.
Benefit: Reduces research costs, speeds up time-to-market for new medications, and improves the success rate of drug development.
Remote Patient Monitoring:
Example: An SLM can analyze data from wearable devices (smartwatches, fitness trackers) and other remote monitoring systems to identify potential health risks (e.g., irregular heart rhythms, sudden weight loss) and alert healthcare providers to concerning changes.
Benefit: Enables proactive healthcare, improves patient safety, and reduces the burden on healthcare systems
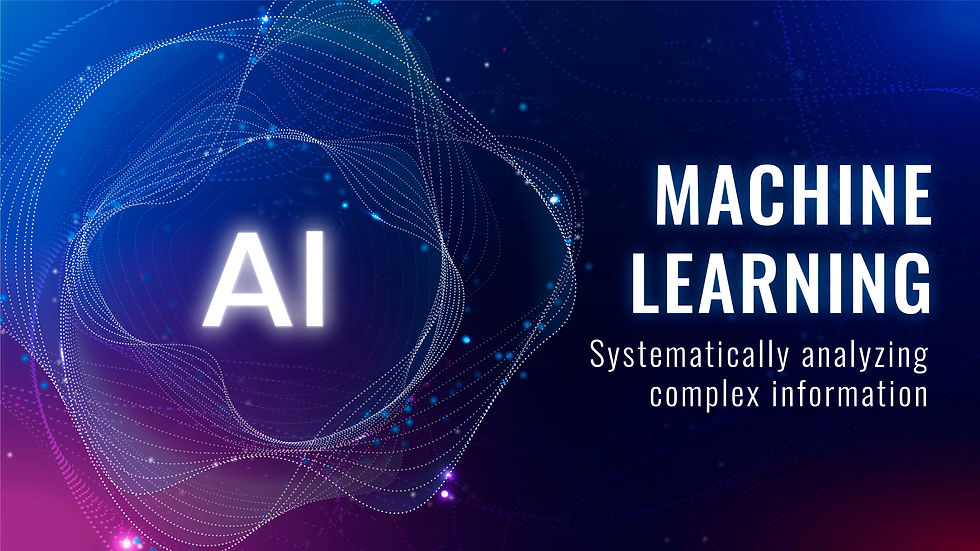
Big Tech's use of Small Language Models (SLMs) in healthcare
Microsoft, Google, and Amazon are all major players in the development and application of Small Language Models (SLMs) in healthcare. Here's a brief overview of their efforts:
Microsoft:
Phi: Microsoft has developed a family of SLMs called "Phi," known for their focus on reasoning and safety.
Potential Healthcare Applications: Phi models could be used for tasks like:
Analysing medical literature: Identifying relevant research, summarising findings, and extracting key information.
Assisting in clinical decision-making: Providing evidence-based support for diagnoses and treatment recommendations.
Developing personalized treatment plans: Analyzing patient data to tailor treatment approaches.
Google:
Gemini: Google's Gemini family of models includes smaller, more efficient versions suitable for resource-constrained environments.
Potential Healthcare Applications: Gemini models could be used for:
Developing mobile health applications: Enabling real-time patient monitoring and remote consultations.
Creating personalized health coaching tools: Providing patients with personalized guidance on diet, exercise, and lifestyle modifications.
Improving the efficiency of administrative tasks: Automating appointment scheduling and medical record management.
Amazon:
Amazon Bedrock: This service provides access to a variety of foundation models, including smaller, more efficient ones.
Potential Healthcare Applications: Amazon Bedrock could be used to power a wide range of healthcare applications, such as:
Developing chatbots for patient support and triage.
Creating tools for drug discovery and development.
Improving the accuracy of medical imaging analysis.
Key Considerations:
Data Privacy and Security: All three companies prioritise data privacy and security in their healthcare AI initiatives.
Ethical Considerations: Ethical considerations, such as algorithmic bias and the responsible use of AI in healthcare, are carefully addressed by these companies.
Regulatory Compliance: All healthcare AI applications must comply with relevant regulations and standards to ensure patient safety and data security.
Nelson Advisors work with Healthcare Technology Founders, Owners and Investors to assess whether they should 'Build, Buy, Partner or Sell' in order to maximise shareholder value > https://nelsonadvisors.co.uk/
Healthcare Technology Thought Leadership from Nelson Advisors – Market Insights, Analysis & Predictions. Visit https://www.healthcare.digital
Buy Side, Sell Side, Growth & Strategy services for HealthTech Founders, Owners and Investors. Email lloyd@nelsonadvisors.co.uk
Nelson Advisors Healthcare Technology Newsletter > Mergers, Acquisitions, Growth, Strategy, Insights & Predictions. Subscribe Today! https://lnkd.in/e5hTp_xb
#HealthTech #DigitalHealth #HealthIT #NelsonAdvisors #Mergers #Acquisitions #Growth #Strategy #Innovation #NHS #VentureCapital #PrivateEquity #UK #Europe
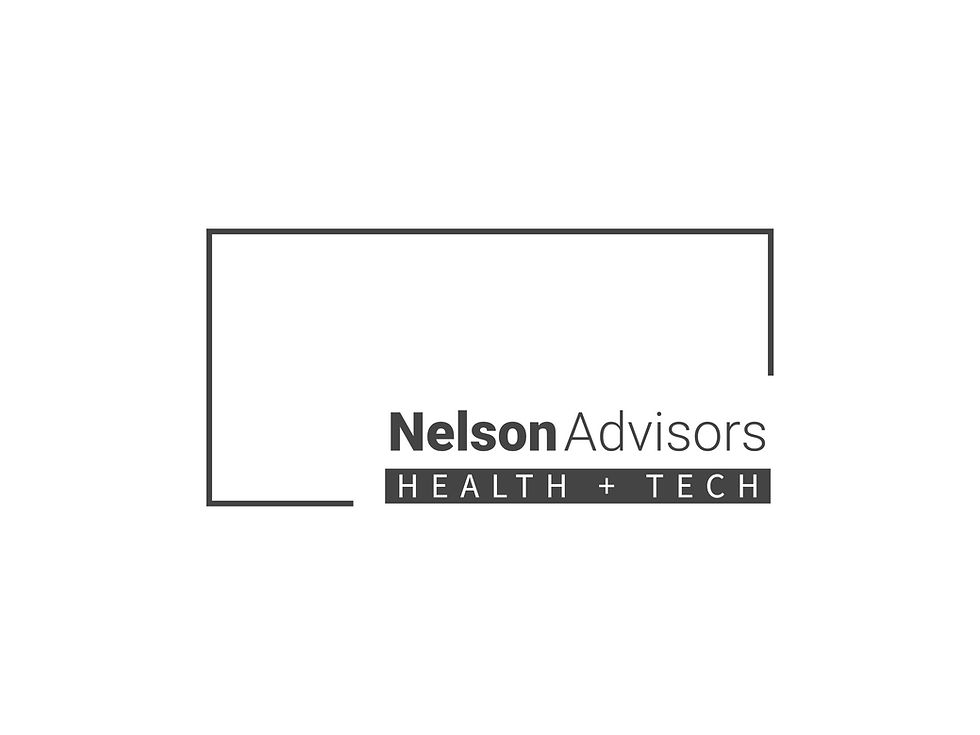
Comentários